Learn about Attribute Enrichment
Great product discovery starts with great product data. However, catalog files often contain incomplete product attribute information. These gaps can present challenges in the search experience as shoppers struggle to find the products they seek, potentially driving them away from a retailer’s website.
Constructor’s Attribute Enrichment engine helps retailers improve their product data automatically. Using best-in-class AI with modern data science methods, it tags your products with new, relevant attributes and categories regularly. Your team can then review these suggestions, which helps the engine generate even better results over time.
Key benefits
- Save time.
- Since Constructor enriches product attributes automatically, you can refocus the time you’d spend on this task elsewhere.
- Reduce mistakes.
- Constructor automates the process of identifying and adding attributes to products, reducing human errors during manual data entry, such as typos, inconsistencies, and omissions.
- Improve customer experience.
- High-quality and relevant product information means a customer is more likely to be satisfied with their purchase and less likely to return it. For example, with precise dimensions for rugs, customers are more likely to choose the one that fits their space.
How Attribute Enrichment works
The attribute enrichment process takes raw product data, such as images, categories, and descriptions, and trains machine learning models to predict and enrich product attributes. Enriched attributes are then ingested into search and browse indexes for improved product discovery and validation.
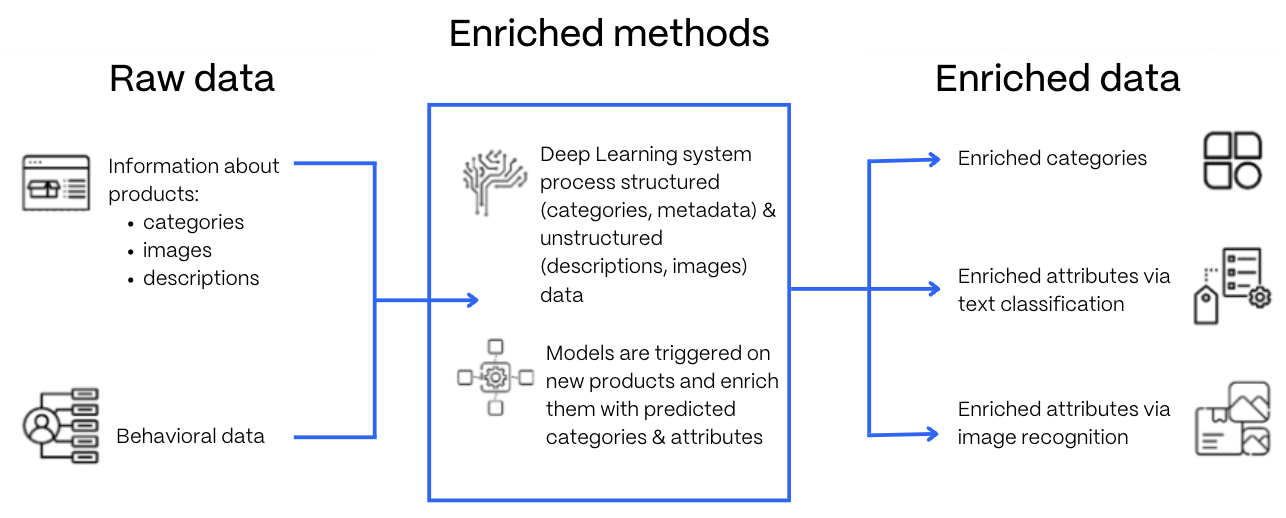
Raw data
We use two types of data as inputs:
- Raw catalog data. This includes whatever product titles, categories, descriptions, and images are present in your catalog file. It can also include variation information like sizes and colors. It’s not uncommon for information to be incomplete or missing.
- Behavioral data. This includes clickstream data from your website, such as search queries, browse filters, shopping sessions, clicks on search results, etc. It helps identify high-demand attributes. For example, if users frequently filter products based on a specific attribute like color or material, then we know that attribute is important.
Enriched methods
When we train the deep learning models, we teach the Attribute Enrichment engine how to make your product information better.
For example, it might involve using product swatch images to identify missing color attributes. It could also involve text enrichment, which extracts attribute information from product names and descriptions (for example: neck size, sleeve length). Multiple methods can be used to train these models.
We also incorporate learnings from clickstream data to pinpoint which attributes to prioritize in the enrichment process based on shopper engagement.
Enriched data
Once identified, enriched attributes are ingested into your search and browse indexes. This is where they begin taking an active role in improving product discovery via search queries and browse experiences. If you wish to, you can export a CSV file with these attributes to upload to your PIM manually.
Enriched attributes also appear in your dashboard where your team can review them (and dismiss them, if necessary). Your team can also leverage enriched attributes to create searchandising rules.
Example enriched product
Let’s look at an example of a product before and after attribute enrichment. Specifically, we’re looking at how the process predicted enriched categories for the product.
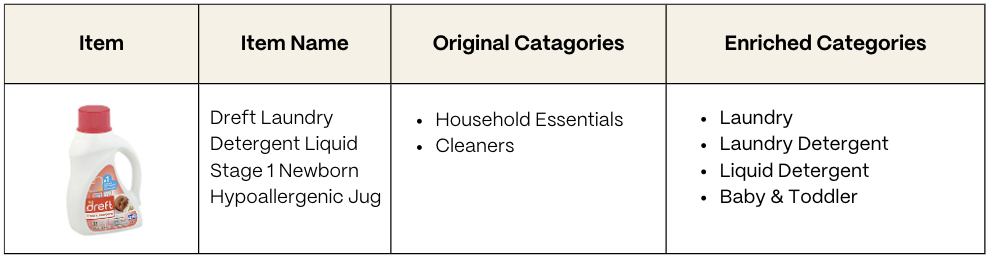
In this example, the product Dreft Laundry Detergent Liquid Stage 1 Newborn Hypoallergenic Jug
had two existing categories in the raw data: Household Essentials and Cleaners.
Through the attribute enrichment process, using models like text enrichment, we predicted additional categories that were relevant for the product: Laundry, Laundry Detergent, Liquid Detergent, and Baby & Toddler.
Adding these categories to the product will improve shoppers' ability to locate the product in categories that are more common or expected for the product.
How to get started
If you are interested in Attribute Enrichment, reach out to your CSM to discuss enabling it. Our team will handle setting up the enrichment pipeline for your operation.
Using Attribute Enrichment
- Review and dismiss enriched attributes. Learn how to review enhanced attributes and dismiss those you deem irrelevant or unnecessary.
- View Attribute Enrichment analytics. Learn how to understand and take action on analytics shown on your Attribute Enrichment dashboard.
Updated 25 days ago